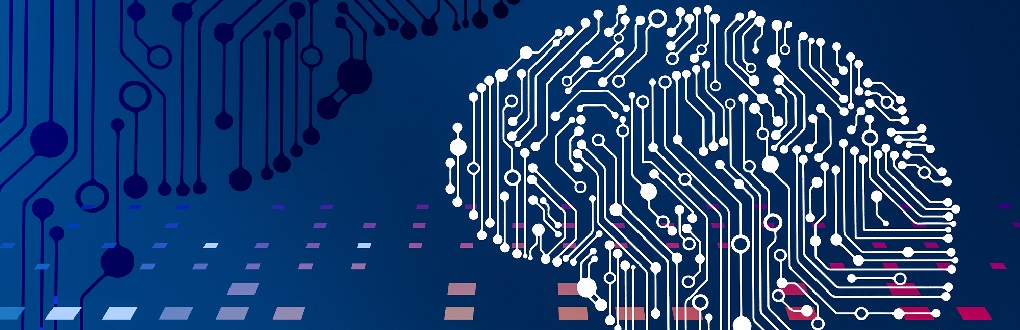
[This is the second in a series of American Society of Journalists and Authors (ASJA) blog posts on artificial intelligence (AI) and nonfiction writers, leading up to ASJA’s AI webinar Wednesday, January 10 from 1:30 to 3:00 ET; free to ASJA members and $20 for nonmembers. Register for it here. The first ASJA blog post on AI provided an introduction to artificial intelligence.]
Is artificial intelligence actually “natural intelligence” in copycat form? Are computers really thinking machines capable of making decisions, acting on them and even sensing the emotions of human operators and trainers?
Well, as Meryl Streep might say, “It’s complicated.”
Freelance journalist and AI explainer Harry Guinness, who will appear at the first ASJA Webinar on AI on January 10, describes AI in blushing terms. “AIs…are able to learn and solve more complex and dynamic problems [than ordinary computer programs] — including ones they haven’t faced before,” Guinness writes. “In the broadest possible sense, artificial intelligence is a machine that’s able to learn, make decisions and take action.”
With caveats, Guinness adds.
For context, it may help to understand the difference between typical computer programs and AI systems like ChatGPT.
While a non-AI computer program is designed to repeat the same task or solve a defined set of problems using pre-structured procedures, an AI system relies on machine learning and natural language processing (NLP), among other tools, to go further.
For example, a sophisticated AI system can recognize and interpret the meaning of large chunks of text. By capturing relationships between individual words and sequences of words in sentences, AI systems discern patterns to predict and generate outputs — text, images, video, audio, software code, equations — whatever a human operator requires.
To accomplish this, the machine trains by crunching through massive data sets, comparing user queries to its knowledge base.The AI system then “learns” by adapting its own algorithms (sets of rules) to classify, analyze and reply to information requests.
Based on its trainings and knowledge base, AI can even interpret conceptual abstractions. For instance, it will calculate the likelihood that specific four-word sequences (coded in AI as “tokens”) follow logically from others. ChatGPT, for one, has been trained on 500 billion tokens extracted from books, articles and billions of pages of content from the internet.
AI programs can also learn to distinguish variable meanings of words, their context and emotional tone (what’s known as “sentiment analysis”). When human trainers offer AI examples, that fine tunes the algorithms to make context clearer and outputs more relevant.
What about Deep Learning?
The “deep learning” part of AI happens when the machine starts learning from itself —modifying its algorithms and predictions without human intervention. This is where AI gets “murky” (Harry Guinness’s word).
That’s because even trained experts don’t understand exactly how AI, parsing through trillions of data points, creates its complex and often weirdly creative (and not always accurate) responses.
The system relies on something known as “artificial neural networks inspired by the human brain,” according to our panelist Linda Whitaker, an expert in operations research and computer science.
“Deep Learning involves the creation of very complex neural networks with many layers and many neurons,” she adds. The construction of [AI-based] neural networks consists of a number of processing “layers,” each containing “nodes” (think of each variable in raw data as a node, received in an “input layer”).
Several hidden layers attempt to learn about different aspects of the data. This is where the ‘black magic’ of AI begins. “Construction of neural networks refers to the number of layers, how the layers are put together, how the nodes process the data and any other specific rules or logic applied,” Whitaker says. Creating interpretable raw data inputs is also key.
To expedite “conversational” outputs in real-world situations, the system invokes Natural Language Processing so it can recognize key words and filter out extraneous verbiage. To humans, AI appears to respond “naturally” even though it’s never encountered the same or similar problems or situations before.
Who Is Hallucinating: AI or Humans?
Can AI be wrong? Yes. Definitely.
AI errors and “hallucinations” have already been common, along with made-up references to non-existent books and articles.
For example, when journalist and fiction writer Vauhini Vara queried ChatGPT about herself for an Op/Ed in The New York Times, the system replied that Vara was a journalist (correct), was born in California (false), received Gerald Loeb and National Magazine awards (both false) and had authored a nonfiction book, Kinsmen and Strangers: Making Peace in the Northern Territory of Australia (false).
Vara’s conclusion: “Trolling a product hyped as an almost-human conversationalist, tricking it into revealing its essential bleep-bloopiness, I felt like the heroine in some kind of extended girl-versus-robot power game.”
Here’s the bottom line: The current state of AI is nowhere near as robust as tech purveyors claim.
The main goal of AI these days isn’t to be right; it’s to keep users engaged while the system trains itself on mistakes and presumably, gets answers right in the future.
Generative AI today (the kind of AI that generates text, image, numbers, video, and maps) is still short of natural intelligence, according to Guinness. “The real win in AI would be to build an artificial general intelligence (AGI) or strong AI…an AI with human-like intelligence, capable of learning new tasks, conversing and understanding instructions in various forms, fulfilling all our sci-fi dreams. Again, this is something a long way off.”
So, what can journalists and content providers do now with AI?
One obvious response is to remain vigilant. Factcheck every AI response. Stay informed about legal cases challenging AI providers on copyright infringement. Rather than taking AI shortcuts to generate news stories, remind editors, publishers and advertisers that human writers remain the only credible source for quality, unique, deeply reported, fact-checked content.
At the same time, it’s wise to remain open to what AI may be able to do both now and in the near future. Some examples:
- Outlining stories and summarizing content to jumpstart the writing process
- Studying publishers’ stories to find patterns in reader behavior and using those patterns to serve readers stories, as Nieman Reports has suggested
- Customizing readers’ experiences by recommending related stories they might like to read
- Translating articles on demand to reach global audiences
- Creating news quizzes
- Generating illustrations, images and infographics by inputting text or data to speeds up content production, especially in smaller newsrooms and marketing organizations with limited resources
- Transcribing and archiving interviews
- Delivering article summaries for faster web dissemination (with final human review of content)
- Delivering readers and subscribers personalized news and individually tailored content based on their preferences and reading habits
- Labeling all AI-generated news feeds, corporate content, and marketing communications to let readers distinguish between human and machine-made content.
Arielle S Emmett earned her Ph.D. at the University of Maryland Philip Merrill College of Journalism. A member of ASJA, she is a contributing editor at Smithsonian Air & Space Magazine and a Fulbright Scholar Kenya (2018-2019). She has also been a visiting faculty member at several universities. Learn more at www.arielleemmett.com.